How Latent Dirichlet Allocation (LDA) Discovers Consumers' Preferences on Coffee Products
- Siti Khadijah Jasni
- Jan 21, 2022
- 3 min read
Machine Learning and Coffee Market: How Latent Dirichlet Allocation (LDA) Discovers Consumers' Preferences on Coffee Products
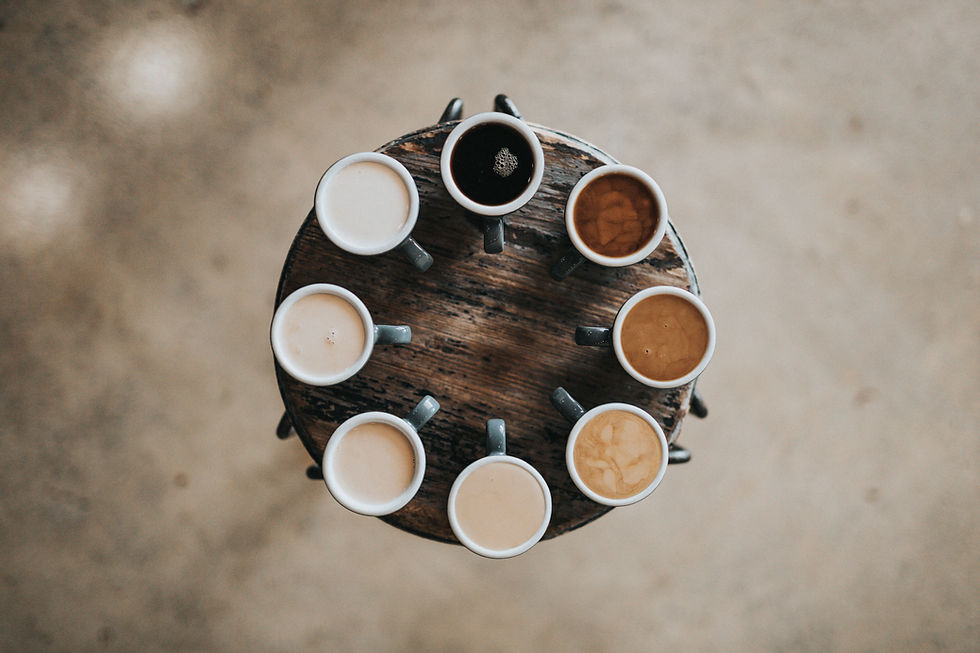
Consumers' online purchases of essential products have drastically changed since the coronavirus pandemic crisis shocked the world. Online platforms such as Amazon.com has started to get high demand in retail, whereby many are increasingly relying on online purchases than ever before. With the increasing purchases of groceries and food products online, obtaining better information has increasingly become crucial to these online platforms to identify factors influencing consumers' choice of online food products.
Thus, understanding how consumers make decisions when shopping online is essential. This situation has made it necessary for Amazon to obtain detailed information on consumers' needs and formulate products that can meet their demands, such as coffee products.
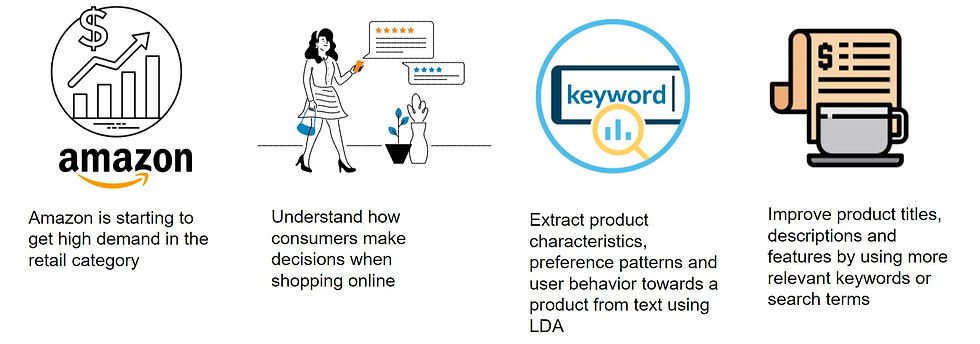
Choosing the right keywords is important to any Amazon sellers to drive traffic to their product listing and move their products to top search rankings. Specifically, Amazon uses A9 algorithms for product search where sellers need to populate as many relevant terms as possible for their listing to show up despite variations and misspelling to increase visibility, sales and overall rank in the search results.
"Based on our experience, keyword order and keyword selection can dramatically affect the sales and ranking of Amazon products."
- Bryan Bowman, bigcommerce.com
Using the plethora of consumers' review information provided by Amazon's online customers of the products they purchase (customer reviews), this study applied a topic-sentiment modelling approach which is Latent Dirichlet Allocation (LDA) and VADER Sentiment Analysis to extract product characteristics and discover consumer preferences and behaviors over 16,275 of coffee products using customer review data and product metadata from Amazon.com.
Apart from extracting product characteristics, choice patterns and consumer behavior towards a product from the text by developing a topic modelling-based method, this project also developed Part of Speech tagging-feature model to improve the accuracy of the LDA model and optimize this model by finding the most relevant number of topics, K.
The overall workflow of the proposed approach is based on both technical and business objectives. This study used Amazon's customer review data and product metadata, consisting of an extensive collection of review texts and product descriptions on grocery and gourmet foods products. Several pre-processing are performed to convert the two raw files into well-structured data. Then, selecting a few attributes that relate to the study's objectives. The raw text file is then converted into a well-defined sequence of linguistically meaningful units.
Then, the LDA algorithm is executed on top of this cleansed dataset to generate product features after extracting words from each review using Part of Speech Tagging (POSTAG). These two steps are run fifteen times based on the POSTAGs combinations. Each model combination is evaluated with topic coherence. The model with the highest coherence score is selected as the final LDA model. Finally, applied VADER Sentiment Analysis to find out whether the product feature is positive, neutral, or negative.
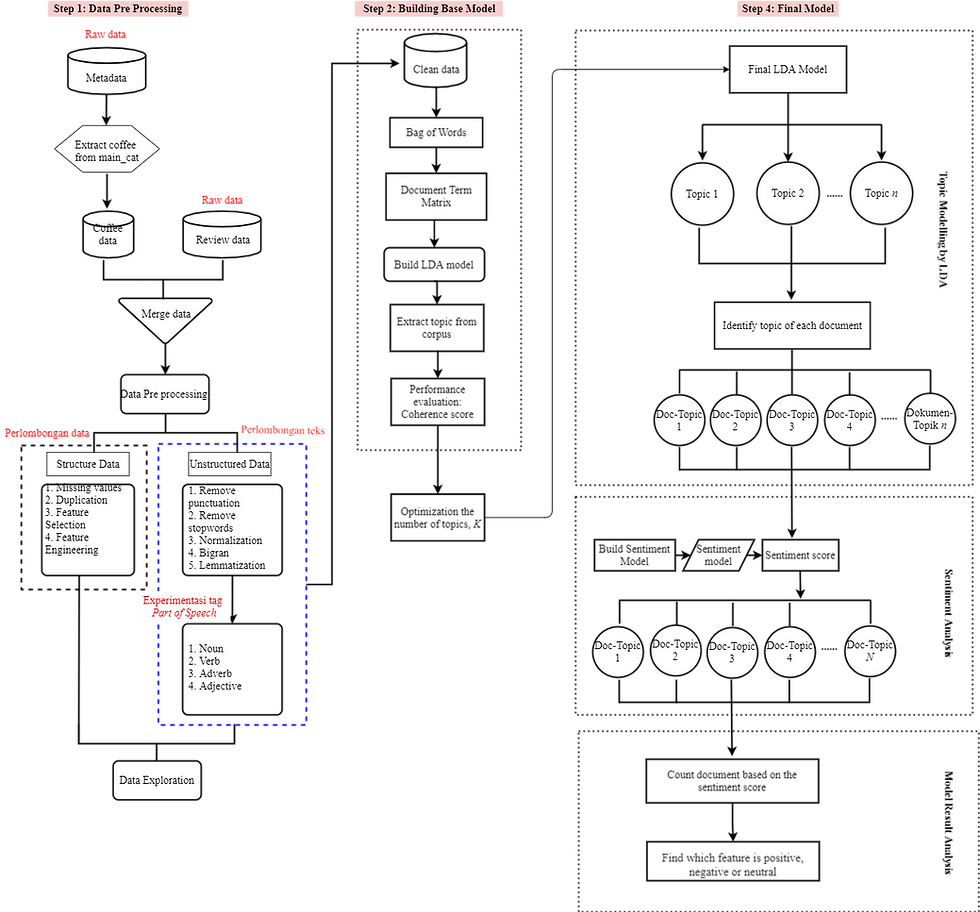
This study found that Nouns, Verbs and Adverbs are the best combinations of word types, and the optimal number of K topics is 6. The characteristics of coffee extracted from this model are Coffee Service, Coffee Quality, Price, Coffee Bean, Coffee Flavor and Packaging. All of these features are positive because positive sentiments have the highest number of reviews compared to negative and neutral sentiments.

To conclude, business managers and sellers can leverage their customers' reviews to better understand their needs by providing better services and to increase their sales by improving the product titles, descriptions and features by using keywords or search more relevant terms. For future recommendations, this project would suggest building an Automatic Topic Labelling to name each of the topics without the need for human judgement.
Comments